#AskTheTaxWhiz: Registering and updating TIN for employees
Apart from these, attempts have been made lately to explore hierarchies as well. Helic D. This paper aims to explore the usefulness of tag hierarchies as directories to facilitate navigation or browsing in social tagging systems. In order to construct such a tag hierarchy, the authors have put forward a new version of an existing centrality based algorithm with a branching factor b as an input parameter which describes the maximum number of categories and sub-categories.
It employs tag co-occurrence as the similarity measure and tag generality as the centrality measure over the tag-tag networks. In a tag network, each tag is considered as a node and is linked to the other node according to a certain occurrence threshold. In the process of building up a hierarchy, the nodes are first ranked in a descending order based on the degree of centrality generality threshold to obtain a centrality list. As a result, the most general tags are placed at the top order. The proposed algorithm has two phase procedure to ensure that as much as the given tags are being connected to the main tree without the tree being fairly deep.
In the first phase, it populates a forest of multiple trees with the most general node as the root node, iterates through the centrality list, identifies the most similar tag to the current tag in the tree computing the co-occurrence threshold and then appends the tag as a child to its most similar tags. It attaches a maximum of subcategories to a given category. Later the produced trees are sorted in descending order of their size no: of categories they possess and the largest tree is considered as the main tree.
In the second phase, the algorithm appends the other trees to the main tree by connecting the root node of a particular tree to the most similar node in the main tree. In case the most similar mode consists of only one free sub-category spot, then a misc category is introduced into the free spot and then the given tree is appended to that misc category. However, the nesting of misc category is also necessary and cannot be avoided completely due to the very structure of tag-tag networks.
Normally, in a typical power-law network, the nodes with high degree centrality are connected to a small number of high and mid degree nodes high centrality as well as to a large number of low degree nodes low centrality. Such high centrality tags occupy the top positions of the hierarchy. Therefore, in the hierarchy building process, the algorithm first appends the adjacent high degree and the mid degree nodes as sub-categories to a given node using up all free sub-category spots followed by the addition of the low degree nodes through the misc category.
It is to be noted that nested misc categories do not affect the semantics of the network but rather keep the tags away from the most related ones into its misc categories. The results and simulation studies illustrates that the proposed algorithm outperforms existing ones in constructing a tag hierarchy useful for better navigation. Maiya and Berger-Wolf [19] introduced a simple and flexible method based on maximum likelihood to infer social hierarchy from weighted social networks.
They have used a simple greedy algorithm to infer maximum likelihood hierarchy from a given network. This approach was evaluated against both simulated as well as real-world datasets for accuracy. This method can also be used to infer the generative interaction models that could lead to a social network. The results show that hierarchies can be inferred from the associations among different entities in a network, provides the frequency and occurrence of theses associations.
Gupte et al. They propose a measure of hierarchy and illustrate how hierarchy and degree of stratification emerge with the increase in network size. The paper presents a measure of hierarchy and a polynomial time algorithm to find the largest hierarchy in directed networks. This paper also shows that with the increase in network size, the size of hierarchy grows significantly but the rate of stratification tends to be slow.
- how to convert vinyl records audio cassetes to mp3;
- The Hierarchy Structure in Directed and Undirected Signed Networks!
- The Science of Growth How Facebook Beat Friendster;
The studies are based on the assumption that there exists a global social rank for every person in a network and each of them is aware of their ranks as well as the ranks of people they link to. It is been observed that when people of higher ranks in a hierarchy links or recommends people of lower ranks there occurs a considerable amount of social agony depending on the difference between their ranks.
In , the problem has been examined and a universal hierarchy measure has recently been put forward by Mones et al. Known as the Global Reaching Centrality GRC , this new measure captures the heterogeneous distribution of local reaching centralities in a network.
LEARNiNG WHiLE EARNiNG:
Unlike other measures so far suggested, GRC claims to overcome many drawbacks and is widely applicable to all classes of complex networks. They propose a universal hierarchy measure based on Global Reaching Centrality and a visualization technique for any type of complex real-world network [22]. In a network, complexity often arises due to the interactions between similar units or as a result of nature of interactions edges and units nodes. Hierarchy is an imperative feature of any complex network.
However, the emergence of hierarchy within a network depends largely on the extent to which a node influences the other as well as the system in whole. In other words, the nodes with a stronger impact can be at a higher order rank in a hierarchy. In fact, determining such nodes becomes crucial in defining a measure of hierarchy. Apart from a tree-like network, a real-world network is much more complicated with the existence of relationships between nodes of the same level, cycles of connected nodes, clusters, edges moving upwards etc. Hence hierarchy detection in networks is very demanding.
Hierarchical measures so far been suggested cannot be applied on different complex systems due to many shortcomings. In order to define a measure based on reaching centralities, the paper essentially focus on flow hierarchies in real and adjustable hierarchical AH networks. The concept of Global Reaching Centrality GRC [21] measures the heterogeneous distribution of local reaching centralities in a graph. In an unweighted directed graph, local reaching centrality is the ratio of number of nodes with finite positive directed distance from a particular node to the maximum number of reachable nodes from the same node.
Therefore, the GRC of an unweighted directed graph can be defined as the difference between the highest and the average local reaching centralities within a network, given by [21] :. For weighted undirected graphs, the generalization of GRC is quite straight- forward based on local reaching centrality as defined for unweighted direct graphs. If there exist more than one directed shortest path from i to j, then the path with maximum weight i.
Similarly, for an undirected unweighted graph, GRC can be obtained by excluding computation of weights of shortest path between two nodes. That is, two nodes chosen, say A and B, are connected if they were not already connected in the AB direction. The p proportion of the edges are connected as AB only if, to preserve hierarchy. Randomization of real networks is done by generating a random network with the same in and out degree with respect to the original model and followed by choosing two random edges AB and CD and then changing the endpoints to obtain AD and CB.
The GRC for an adjustable hierarchical AH network is found to change continuously and monotonously in an interval of a highly random state to a fully hierarchical one. In the case of real networks, the edges are directed so that the origin of the edge has a greater impact on terminal. It has been observed that GRC depends largely on the average degree and network structure. A network with higher average degree has a smaller GRC indicating the existence of a lower hierarchy.
However, the comparison of the actual GRC value with GRC of the randomized versions of the original networks exhibits slight variations.
Browse by Content Type
In order to analyze the correlation between hierarchy and controllability of a network, GRC is then compared with the number of driver nodes under switch board dynamics. Here, driver nodes are nodes that control the state of every edge. For a total control over an easily controllable network, the no: of driver nodes to be controlled are few.
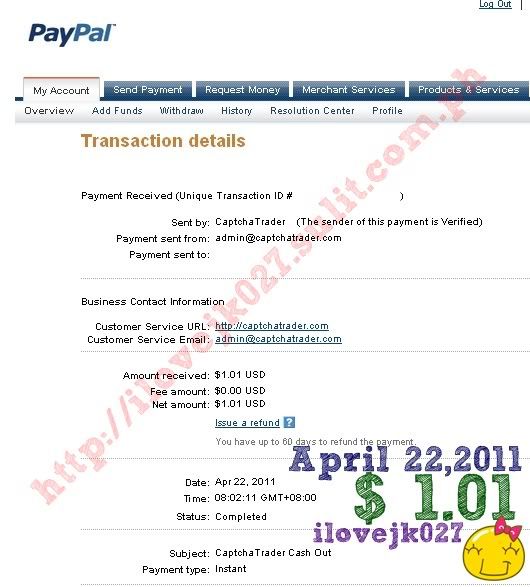
The results so obtained tend to exhibit a negative correlation between the two quantities, i. GRC and are inversely proportional to each other. This clearly suggests that a hierarchical network is better controllable. The proposed hierarchical visualization technique for large graphs assigns each node into different levels on the basis of a local quantity. For an unweighted digraph this local quantity is equal to the local reaching centrality.
Therefore, an ER graph posses a two layered hierarchical structure and arborescence has many layers. The structure of an SF graph lies in between an ER graph and an arborescence with a few clearly separated layers. To avoid different hierarchical lay-outs for single graphs of same graph model, ensembles of ER, SF, directed AH and real networks are visualized.
In short, the proposed hierarchy measure, GRC quantifies the heterogeneity of local reaching centrality in whole network by introducing bidirectional edges among equivalent nodes. It is free from the drawbacks of the hierarchy measures so far been suggested. Hence, it can be concluded that GRC is a more suitable measure for hierarchy in any network. Examples could be road network, world-maritime network etc. Social network, where people represent nodes and the relationship between them represents links. A Link can be either directed e.
Facebook where relationship is mutual. Physical networks like Road network, World maritime network.
Browse by Subject
Here nodes are represented by Cities and Ports respectively, and links are represented by routes. Biological networks: One of the examples is Protein-Protein interaction network where each protein is a node and interaction between them is represented by a link. Among all the networks our focus is mainly on social networks.
It shows some different properties in compare to other networks present like internet, World Wide Web. A network can be categorized into Infrastructure network and Interaction network. In infrastructure networks a link can be established even if the nodes do not interact with each other. Typical examples of infrastructure networks are Facebook, Orkut, Twitter, and etc.
In Interaction network a link is setup among nodes when they interact with each other.
- Feed aggregator;
- IS IT AFFORDABLE TO ORDER CUSTOM ESSAYS??
- how to get birth certificate from germany;
- Post navigation?
- Hit Me More.. :)?
- Accounts & Passwords?
- find out who called your cell phone;
Typical examples are Protein-Protein interaction network, Slashdot social network which is a result of interaction between nodes due to the threaded discussion. One major difference between the two networks is a link may lose its importance during the course of time in Infrastructure network i. For example, if one does not interact with a person on a regular basis then the link which connects both of them loses its importance with time.
But in interaction network a link never loses its importance with time, as the nodes continue to interact with each other regularly.
Professional Custom Essay Writing Service - Get the Best Essays Here
A network can also be classified as signed and unsigned networks. Online social networks like Facebook, twitter, friendster, and etc come under this category. Both signs can be interpreted differently in different networks. Studies so far reveal only certain typical statistical properties shared by most of the complex networks. Some of distinctive properties include small-world phenomena [24] , power-law degree distributions [25] , clustering also called as network transitivity [26] , community detection [22] etc. However, there still remain certain issues that are open.
Hierarchy being one such issue has attracted many scientists.